The widespread use of AI poses numerous challenges. Including the risks of data leakage, the need for explainable results, handling it in SaaS. But also, the growing dependence on Big Tech. Not to mention the environmental toll linked to AI.
No doubt the eco-design of digital services is becoming increasingly popular. Still, the efforts to achieve digital sobriety seem to be marginal. Especially compared to the energy consumed by training general-purpose LLMs. Is there a way to make AI greener? And what would a more “trusted AI” mean?
Here’s a roundup of challenges and solutions.
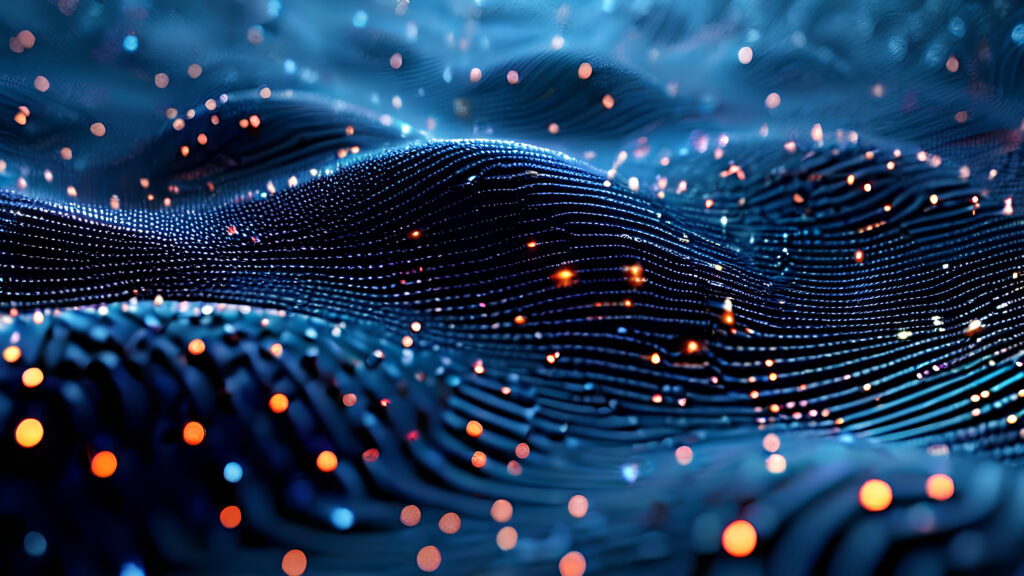
Efficiency of specialised LLMs compared to general-purpose LLMs
General-purpose LLMs, such as GPT-4 developed by OpenAI, LaMa (Meta) and Gemini (Google) are currently in the spotlight. Versatile, omniscient, and able to handle a variety of scenarios. They seem to be able to meet every need: generating text, code, answering questions, translating content, and even composing poems.
However, these general-purpose models have not yet eclipsed specialised LLMs,[1] which target a narrower range of situations, but perform much better in them. The Retrieval-Augmented Generation (RAG) technique certainly makes it possible to specialise a general-purpose LLM via transfer learning, with or without retraining the model. However, the use of general-purpose LLMs continues to pose a range of challenges. Starting with their generic results, unreliable quality or lack of reproducibility. This will prove even more challenging as the available sources of quality data may become scarce, due to legal actions[2] brought for unauthorised use of content and copyright infringement. Additionally, the use of general-purpose LLMs leads to operator dependency and reinforce monopolies,[3] which is unfavourable for long-term users.
The impact of AI on the environment
Researchers from Hugging Face and the Allen Institute[4] have shown that in the case of servers with GPUs, the carbon emissions linked to machine use far exceed those linked to manufacturing the components, unlike traditional cloud computing. Generating an image using an AI model is one of the most energy-intensive uses, and requires as much electricity as fully charging a smartphone.[5] Reversing the distribution of carbon emissions throughout the lifecycle of servers in this way means that the power usage effectiveness (PUE) of the datacentres in which AI models are trained and inferred, as well as the energy mix of the countries in which they are located, are very significant selection criteria in calculating your application’s global carbon footprint.
This is a bonus for OVHcloud. Indeed, the Group has long been committed to reducing the carbon footprint of its datacentres.[6]
As it might be expected, general-purpose LLMs are more environmentally damaging than specialised models designed for specific tasks. This has been revealed in a series of comparative tests carried out by the same researchers.[7] With thousands of billions of settings, the largest LLMs are getting larger and more data-intensive.[8] An article in the New Scientist recently explained that algorithm advances are outpacing Moore’s Law, as after eight months. A large language model would need only half the computing power to achieve the same level of performance.[9] However, to run a model like OpenAI today, it would cost Microsoft around $700,000 per day[10], or an average cost of 36 cents per query. Still unreasonable from an economic and environmental point of view to meet needs that are often precise and well-defined.
Specialised models, which can be chained to perform complex tasks (referred to as agentisation), are therefore a more environmentally responsible alternative to general-purpose LLMs. On top of that, specialised models, which are more widely available in open source, are also easier to understand and to fine-tune. They seem more suitable for reversibly building innovations for which the ROI is still very uncertain.
Maintaining control: working towards developing a trusted AI
While large companies quickly became aware of the risks of leaking confidential data when using digital services (like online translation, which they are beginning to ban), AI intensified the temptation to output a company’s data and submit it to an algorithm: here to write a report more quickly, there to generate an image that will illustrate a presentation on a confidential project. Samsung learned this the hard way, as a victim of three consecutive data leaks related to the use of ChatGPT by its employees, who notably copied/pasted source code to solve or optimise a problem.
You don’t need to disclose a lot of information to say a lot about your intentions. What insights would your rival gather about your strategy from reading your ChatGPT prompts? After all, it is possible for AI to accidentally “scrape” data submitted by users, thanks to a bug[11] causing security issues. The same goes for datasets you might submit on AI platforms: will your data be used to train and refine the model? Could they benefit potential rivals?
Beyond this, there is also the question of the transparency of AI models. With it, comes the risk of outsourcing increasingly important tasks to sophisticated AI models. Indeed, they can become “black boxes”, making incomprehensible decisions, or producing skewed results because of the data they are trained on.
Let’s face the possibility that you may not have any problem with the results. Would you run the risk of relying on a service where you can’t explain in broad terms how it works? And that you couldn’t stop using without losing everything? Here, we encounter another problem – reversibility.
If for example, the AI service deemed the party to be over and the infrastructure that it has long financed at a loss must now be made profitable, so it takes advantage of its monopoly and your dependency to increase its rates in an unreasonable way, you could certainly cancel the service. But then you would lose the results of your data training and/or model specialisation, and you would have to start from scratch. In the current absence of standards for portability/interoperability between different AI services, this issue is crucial – all the more so given that, for the moment, while open-source is popular, proprietary models are very much in the majority.
There is no simple answer to the questions that have been raised. That’s because AI development is currently very empirical, based on a trial-and-error model, with no traceability of training data or model modifications.
This, incidentally, makes the “explainability”[12] of an AI system’s results a real challenge, even though the AI Act establishes a duty to do so (see below).
The development of a “trustworthy AI”, as it was termed in a 2019 paper[13] by the Independent High-Level Expert Group on Artificial Intelligence (AI HLEG), is perhaps a direction to keep in mind. It defines a trustworthy AI with three main objectives, which OVHcloud aims to help you achieve: AI must be lawful (legislative or regulatory aspect), ethical (respect for ethical norms) and robust (from both a technical and social perspective).
In the meantime, ensuring swift regulatory compliance at the national, European, and international levels is a powerful lever for promoting greener business practices, without compromising future prospects in the pursuit of innovation.
1/ Complying with current and future regulations
The EU was quick to respond to the democratisation of AI, proposing a draft European regulation on the subject on 21 April 2021. In March 2024, the AI Act was officially adopted. Now, it applies to all services used in the EU, regardless of whether the providers are foreign or not.
The law divides AI systems into four categories, taking into account their impact on fundamental rights in the EU and the security of individuals, groups, societies, and civilization. Each risk category has associated prohibitions[14] and obligations, ranging from environmental sustainability to security, and including marking content that has been AI-generated.
A “compliance checker” online allows you to quickly find out the extent to which this European AI law applies to your projects: https://artificialintelligenceact.eu/
Other national and European regulations on personal data protection, such as the GDPR, already apply to your AI projects, holding companies accountable for hosting and transferring personal data outside the EU.
Incidentally, those who complain about regulation being too burdensome in comparison to the American laissez-faire attitude or the Chinese spirit of conquest have the wrong end of the stick: the absence of a genuine European single market is a much bigger factor[15] behind Europe’s innovation gap. So, too, is the weak support for public procurement, or the incomprehensible message sent by governments that claim to want to develop sovereign solutions by relying on investments by foreign stakeholders.[16]
It’s also worth noting that the AI Act provides for the possibility for national competent authorities (the ICO in the UK) [1] to set up “regulatory sandboxes”, i.e. a controlled environment to test innovative technologies for a limited time in order to ensure the compliance of the AI system and to not delay any potential placing on the market, with priority access to these sandboxes for SMEs and startups.
In short, regulations today do not hinder the development of projects that take advantage of the possibilities offered by AI, but rather strengthen companies’ obligations regarding the protection of personal data due to increased risks. These obligations will help to reassure users, once this brief period of carelessness and frivolity with AI has passed, and the inevitable first scandals start to surface. As Yoshua Bengio, researcher and founder of MILA (the Quebec Artificial Intelligence Institute), summed up: “We’re going too fast in an unfamiliar direction, and that could change the world in a very positive, or very dangerous, way.”[17] Countries should therefore seek to regulate AI so that its development does not feel like the Wild West.
In this context, the preference for sovereign solutions will make it easier for your projects to comply with regulations, in addition to establishing a clear medium- and long-term vision. COVID and the current geopolitical instability have shown the cost of relying on foreign entities for essential services, and AI-based services will quickly follow the same path if they integrate the software we use every day, in such critical areas as health, education, transport, or logistics.
[1] General-purpose and specialised LLMs can be distinguished by the number of parameters in their neural network: tens, hundreds or even thousands of billions of parameters for a general-purpose model versus “a few billion” for a specialised model.
[2] https://www.usine-digitale.fr/article/openai-cible-par-deux-class-actions-aux-etats-unis.N2148412; https://www.lefigaro.fr/secteur/high-tech/des-journaux-americains-poursuivent-openai-et-microsoft-en-justice-pour-violation-de-leurs-droits-d-auteur-20240430
[3] https://www.nytimes.com/2024/06/05/technology/nvidia-microsoft-openai-antitrust-doj-ftc.html
[4] http://arxiv.org/pdf/2311.16863
[5] https://www.technologyreview.com/2023/12/01/1084189/making-an-image-with-generative-ai-uses-as-much-energy-as-charging-your-phone/
[6] https://corporate.ovhcloud.com/en-gb/sustainability/environment/
For our PUE calculation methodology, refer to https://corporate.ovhcloud.com/sites/default/files/2024-01/methodo_carboncalc_0.pdf
[7] https://www.silicon.fr/llm-generaliste-specialise-angle-environnemental-473911.html
[8] https://www.radiofrance.fr/franceculture/podcasts/le-journal-de-l-eco/le-cout-environnemental-de-l-ia-est-colossal-et-sous-evalue-3781962
[9] https://www.newscientist.com/article/2424179-ai-chatbots-are-improving-at-an-even-faster-rate-than-computer-chips/
[10] https://usbeketrica.com/fr/article/chatgpt-coute-t-il-vraiment-700-000-dollars-par-jour-a-openai
[11] https://arstechnica.com/information-technology/2023/02/chatgpt-is-a-data-privacy-nightmare-and-you-ought-to-be-concerned/
[12] https://www.cnil.fr/fr/definition/explicabilite-ia
[13] https://op.europa.eu/en/publication-detail/-/publication/d3988569-0434-11ea-8c1f-01aa75ed71a1
[14] AI systems are prohibited if they violate EU values by infringing on fundamental rights, such as:
• Subliminally manipulating behaviours
• Exploiting individuals’ vulnerabilities in order to influence their behaviour
• AI-based social scoring used by governments for general purposes
• The use of “real-time” remote biometric identification systems in publicly accessible spaces for law enforcement purposes (with exceptions).
[15] https://twitter.com/hubertguillaud/status/1795001082843713968
[16] https://twitter.com/canardenchaine/status/1795862230782640367
[17] https://ici.radio-canada.ca/ohdio/premiere/emissions/ils-ont-fait-annee/segments/entrevue/469120/robot-chatgpt-lois-securite-ordinateurs
To be localised in translation
Germain, with over 20 years at OVHcloud, has held key roles in IT infrastructure, digital transformation, and sustainability. Notable positions include VP of Network Engineering and Head of Bare Metal. He led OVHcloud's North American expansion (2012-2015) and now heads Marketing for AI solutions, focusing on collaborations with startups and partners to advance OVHcloud's AI initiatives.